The Air Quality Modelling group focuses on enhancing the current understanding of atmospheric composition and its evolution. We synthesise weather prediction, dispersion, chemistry transport, and reduced complexity models, which can be employed to comprehend the intricate atmospheric processes influencing air quality over a region. The group utilises a variety of ground- and space-based atmospheric measurements to validate model outcomes. By harmonising diverse models and observations, we aim to provide insights for formulating effective air pollution control strategies within a specified geographical area.
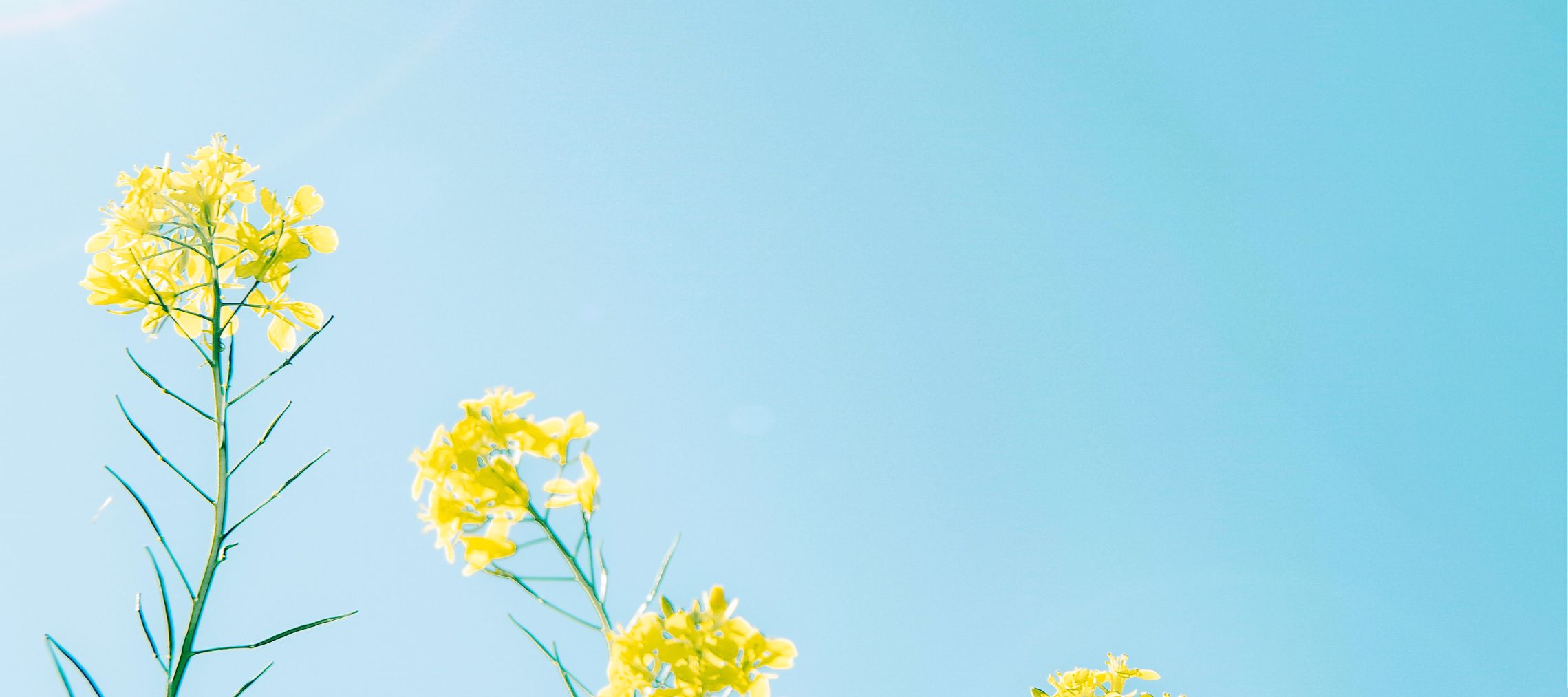
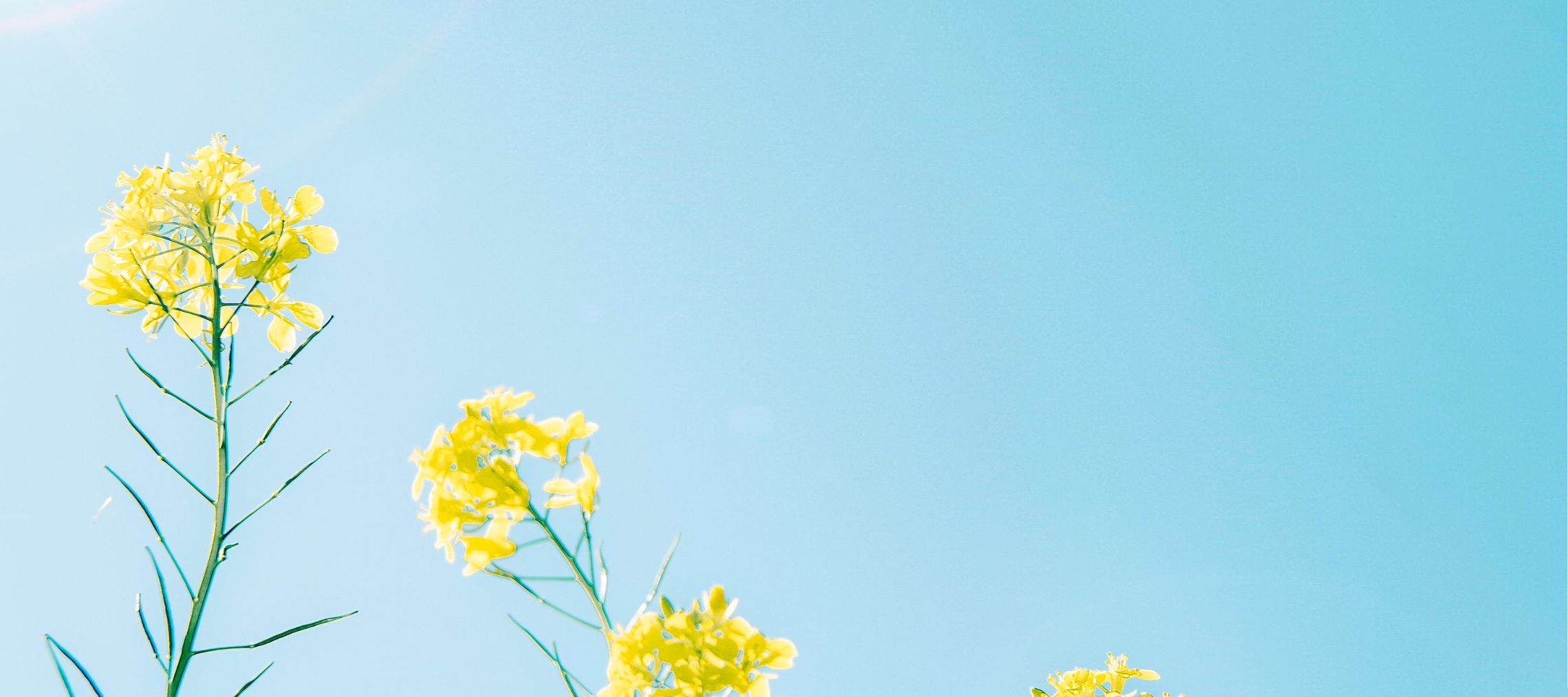
Workshop on the Fundamentals of Air Quality Modelling and Its Role in Air Quality Management
CSTEP organised a two-day regional workshop on ‘The Fundamentals of Air Quality Modelling and Its Role in Air Quality Management’ at Inspire Hall, Le Méridien New Delhi, with support from Open Philanthropy and Bloomberg Philanthropies.
Date: 1.4.2025 to 2.4.2025
The diesel generator dilemma: Reliable power, unwanted emissions
If you face frequent power outages in your area, diesel generators (DGs) are possibly your only saviour during such times. They are known to provide reliable and uninterrupted power supply, which is especially needed in rural regions or areas with limited access to the grid. DG sets are an important tool, particularly for sectors such as telecommunications and healthcare.
But have you wondered about their emissions and how harmful they are to humans and the environment?
Analyst/Senior Analyst
For this post, we are seeking candidates with a background in atmospheric sciences, air pollution, etc., with proficiency in FORTRAN, shell scripting, and python on high-performance computing platforms based on Unix/Linux. The applicant should have experience of working with satellite datasets (e.g., MODIS, TROPOMI, etc.) and surface measurements such as CPCB CAAQMS/NAMP and sensor data. She/he should be able to perform data analysis and visualisation with a strong understanding of statistical methods.
Senior Associate
For this post, we are seeking candidates with expertise in weather/chemistry transport modelling, and satellite data analysis and visualisation. The applicant must have experience of working with Weather Research and Forecasting model with Chemistry (WRF-Chem) and/or Comprehensive Air Quality Model with Extensions (CAMx) on High-Performance Computing (HPC) platforms.
Introducing the CSTEP Air Quality sector
Air quality can be improved through well-designed air quality management plans that are effectively implemented. For this, we need to know the sources impacting a certain location; the emissions contributed by each source; the interventions that can reduce these emissions; the cost of those interventions; and once implemented, their effectiveness. The sources in question can be local (e.g., city traffic emissions), transboundary (e.g., an industry outside a city or sources in another state), or natural (e.g., desert dust or wildfires).