Our work encompasses the analysis of ambient air pollutants using reference- or research-grade instruments and low-cost sensors. We go beyond PM mass concentrations to investigate aerosol composition with state-of-the-art filter-based laboratory analysis and in-situ instrumentation. Our expertise in the proper, science-guided use of these techniques is obtained by conducting field campaigns, source apportionment studies, and systematic evaluations of low-cost sensors, as well as literature reviews. We share this knowledge with the wider community through capacity-building workshops, data portals, and scientific publications. Our data are used to inform policy and support model development.
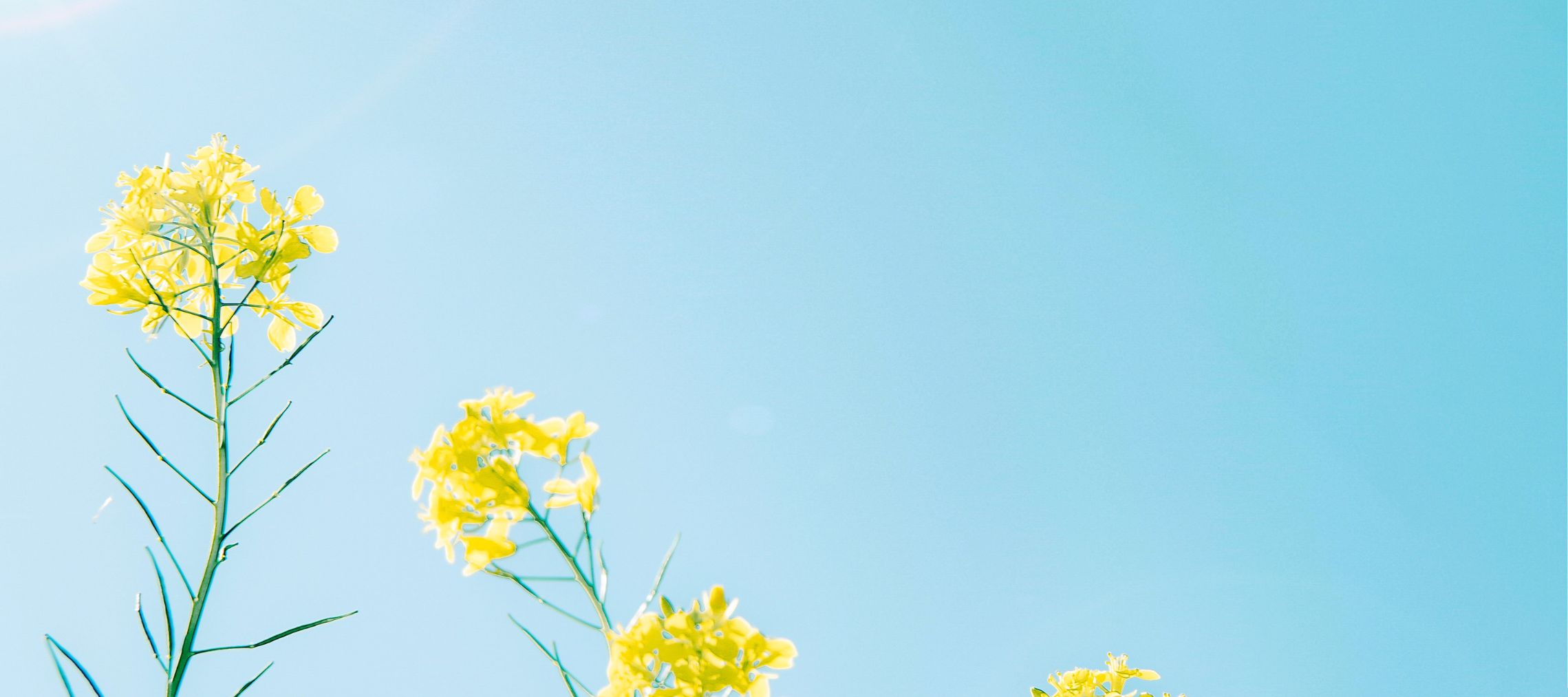
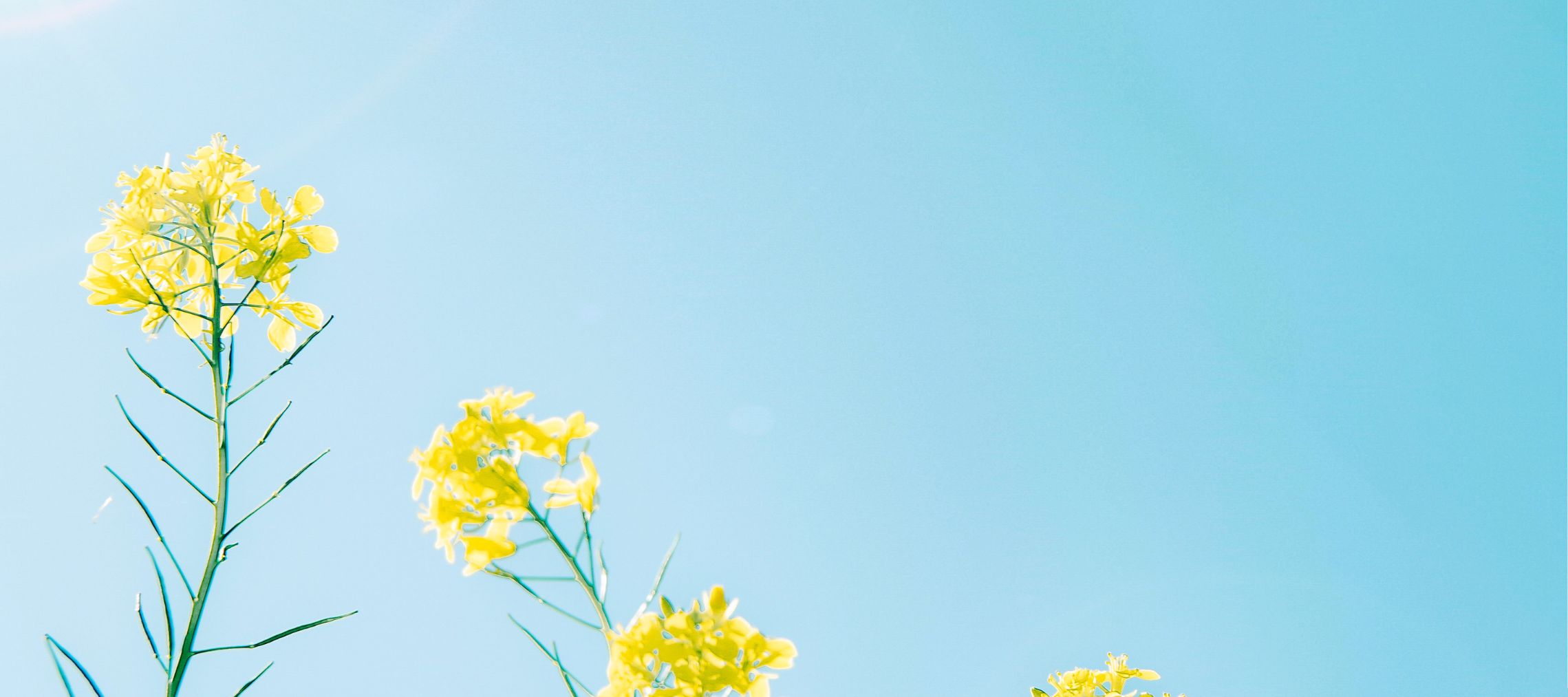
Urban air-quality estimation using visual cues and a deep convolutional neural network in Bengaluru (Bangalore), India
Mobile monitoring provides robust measurements of air pollution. However, resource constraints often limit the number of measurements so that assessments cannot be obtained in all locations of interest. In response, surrogate measurement methodologies, such as videos and images, have been suggested. Previous studies of air pollution and images have used static images (e.g., satellite images or Google Street View images).
Application of an Ultra-Low-Cost Passive Sampler for Light-Absorbing Carbon in Mongolia
Low-cost, long-term measures of air pollution concentrations are often needed for epidemiological studies and policy analyses of household air pollution. The Washington passive sampler (WPS), an ultra-low-cost method for measuring the long-term average levels of light-absorbing carbon (LAC) air pollution, uses digital images to measure the changes in the reflectance of a passively exposed paper filter. A prior publication on WPS reported high precision and reproducibility.
Long-term trend of PM2.5 over five Indian megacities using a new statistical approach
PM2.5 is one of the major contributors to air pollution in India, resulting in poor air quality and human health issues. The current study explores the applicability of TTAinterfaceTrendAnalysis (an ‘R’ language-based Graphical User Interface package) in estimating the annual and month-wise trends in PM2.5 over five Indian megacities (New Delhi, Kolkata, Mumbai, Hyderabad, and Chennai). The package offers a one-stop solution for various statistical analyses such as data imputation, outlier detection, trend analysis, data smoothing, diagnostic tests, etc.
The International Global Atmospheric Chemistry project comments on the revised WHO air quality guidelines
In 2021, the World Health Organization (WHO) air quality guidelines were revised for six key air pollutants. The new guideline levels for PM2.5 and O3 may be unattainable for many countries due to natural sources and background levels. Reductions in NO2 may increase O3 due to atmospheric chemistry. Regional-specific scientific expertise is necessary to guide local air quality management towards more appropriate WHO interim targets and controls on modifiable factors especially considering atmospheric chemistry.
Senior Associate/Research Scientist
We are seeking early-career or aspiring scientists with expertise in atmospheric chemistry and air quality measurements and interest in air quality policy. The candidate should have experience in using AMS/ACSM or similar higher-end instruments or in deploying and analysing data from large networks of low-cost sensors for characterising urban and regional air pollution. Familiarity with air quality management will be a plus.
PRESS RELEASE - Mapping Bengaluru’s air pollution using hybrid monitoring methods
The study conducted by the Center for Study of Science, Technology and Policy (CSTEP) employed a hybrid measurement approach to generate high-resolution air pollution maps. A mobile monitoring campaign was conducted where various monitoring instruments were set up in a CNG-fuelled car, which covered approximately 10% of the city's roads, to measure on-road mass concentrations of fine particulate matter (PM2.5), black carbon (BC), and number concentrations of ultrafine particles (UFPs).
Multiple PM Low-Cost Sensors, Multiple Seasons’ Data, and Multiple Calibration Models
In this study, we combined state-of-the-art data modelling techniques (machine learning [ML] methods) and data from state-of-the-art low-cost particulate matter (PM) sensors (LCSs) to improve the accuracy of LCS-measured PM2.5 (PM with aerodynamic diameter less than 2.5 microns) mass concentrations. We collocated nine LCSs and a reference PM2.5 instrument for 9 months, covering all local seasons, in Bengaluru, India. Using the collocation data, we evaluated the performance of the LCSs and trained around 170 ML models to reduce the observed bias in the LCS-measured PM2.5.
Mapping air pollution in Bengaluru using low-cost sensors and mobile monitoring data
To effectively manage air pollution, we need to measure it accurately and at high spatial resolution. However, maintaining a dense network of regulatory instruments is financially and technically burdensome for low- and middle-income countries. A hybrid approach that combines non-conventional, less expensive, short-term stationary, and mobile deployments may be a cost-effective solution. In the city of Bengaluru, India, we adopted such a hybrid measurement approach to generate high spatial resolution air pollution maps.