In this study, we combined state-of-the-art data modelling techniques (machine learning [ML] methods) and data from state-of-the-art low-cost particulate matter (PM) sensors (LCSs) to improve the accuracy of LCS-measured PM2.5 (PM with aerodynamic diameter less than 2.5 microns) mass concentrations. We collocated nine LCSs and a reference PM2.5 instrument for 9 months, covering all local seasons, in Bengaluru, India. Using the collocation data, we evaluated the performance of the LCSs and trained around 170 ML models to reduce the observed bias in the LCS-measured PM2.5.
Types
Journal Articles
Caption
Multiple PM Low-Cost Sensors, Multiple Seasons’ Data, and Multiple Calibration Models
Upload Documentations
From Date
To Date
status
Live
Image
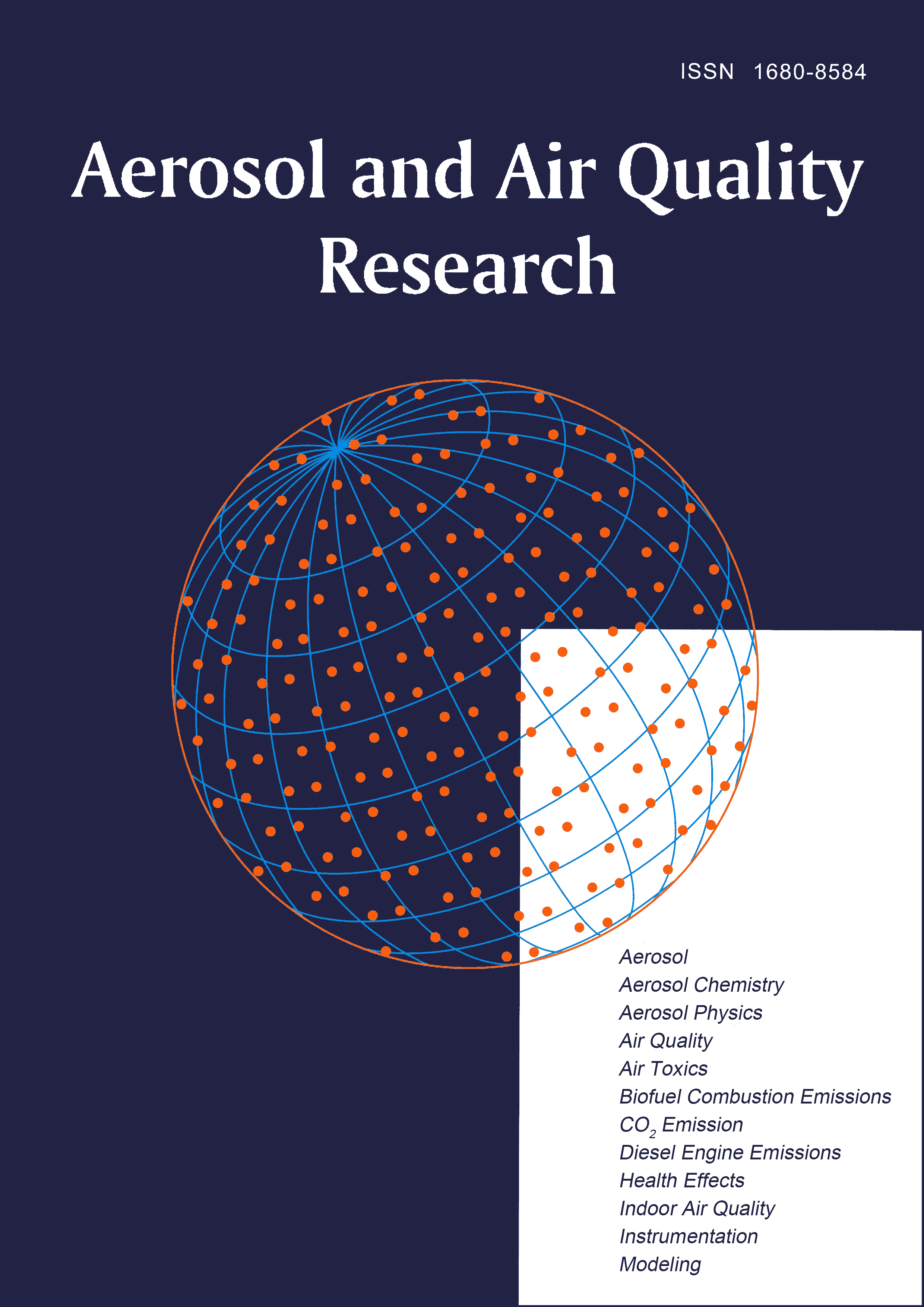
Published by
Aerosol and Air Quality Research
Description (Below Key Messages)
Publication Detail Header Image
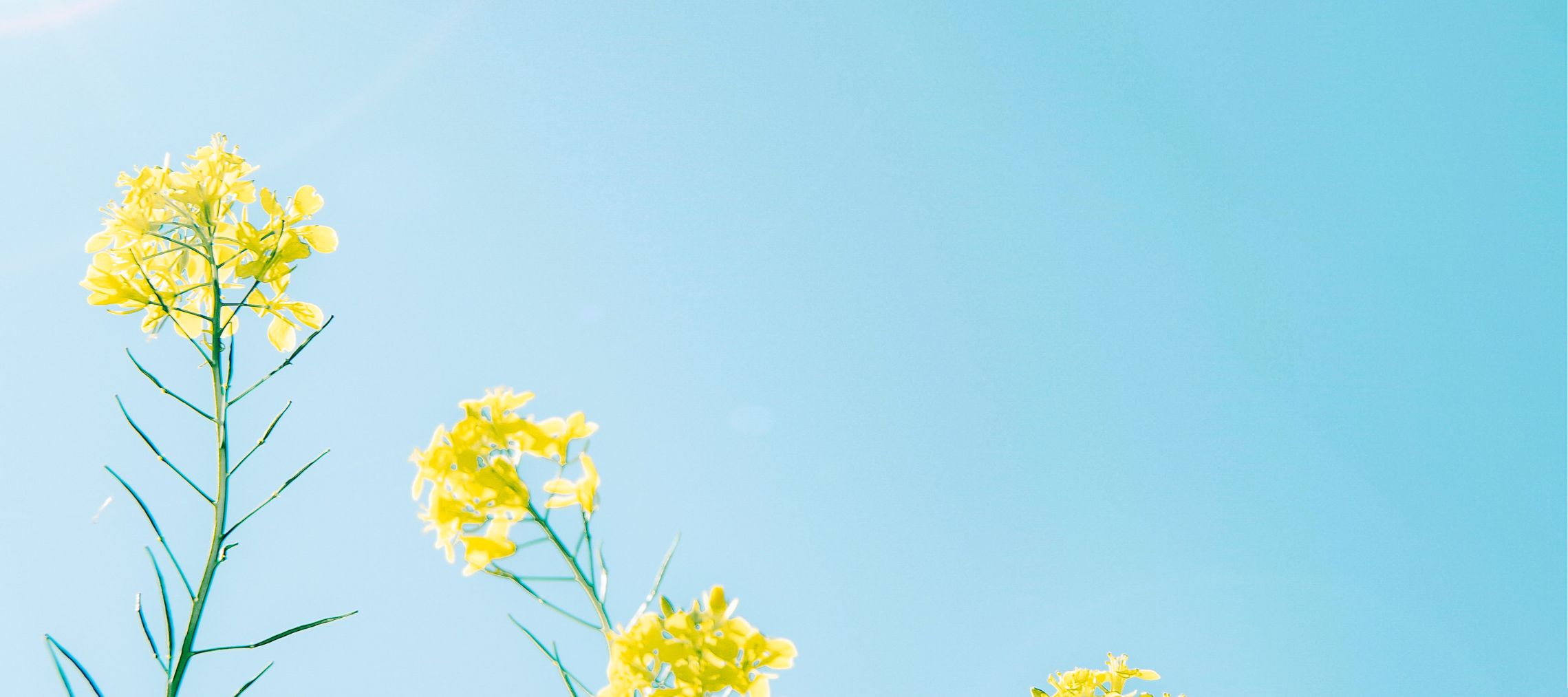